global_gather¶
- paddle.distributed.utils. global_gather ( x, local_count, global_count, group=None, use_calc_stream=True ) [源代码] ¶
global_gather根据global_count将x的数据收集到n_expert * world_size个expert,然后根据local_count接收数据。 其中expert是用户定义的专家网络,n_expert是指每张卡拥有的专家网络数目,world_size是指运行网络的显卡数目。
如下图所示,world_size是2,n_expert是2,x的batch_size是4,local_count是[2, 0, 2, 0],0卡的global_count是[2, 0, , ], 1卡的global_count是[2, 0, ,](因为篇幅问题,这里只展示在0卡运算的数据),在global_gather算子里, global_count和local_count的意义与其在global_scatter里正好相反, global_count[i]代表向第 (i // n_expert)张卡的第 (i % n_expert)个expert发送local_expert[i]个数据, local_count[i]代表从第 (i // n_expert)张卡接收global_count[i]个数据给本卡的 第(i % n_expert)个expert。 发送的数据会按照每张卡的每个expert排列。图中的rank0代表第0张卡,rank1代表第1张卡。
global_gather发送数据的流程如下:
第0张卡的global_count[0]代表向第0张卡的第0个expert发送2个数据;
第0张卡的global_count[1]代表向第0张卡的第1个expert发送0个数据;
第1张卡的global_count[0]代表向第0张卡的第0个expert发送2个数据;
第1张卡的global_count[1]代表向第0张卡的第1个expert发送0个数据。
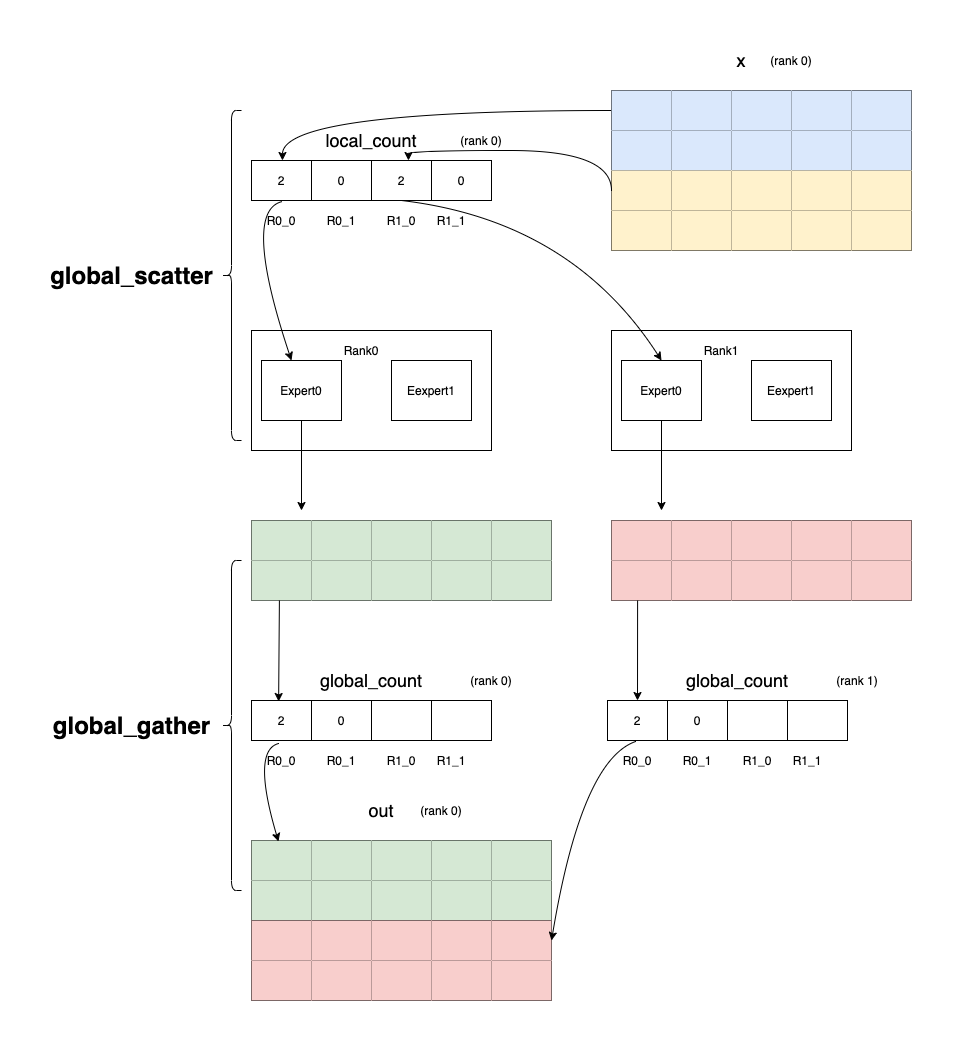
参数¶
x (Tensor) - 输入Tensor。Tensor的数据类型必须是float16、float32、 float64、int32、int64。
local_count (Tensor) - 拥有n_expert * world_size个数据的Tensor,用于表示有多少数据接收。Tensor的数据类型必须是int64。
global_count (Tensor) - 拥有n_expert * world_size个数据的Tensor,用于表示有多少数据发送。Tensor的数据类型必须是int64。
group (Group, 可选) - new_group返回的Group实例,或者设置为None表示默认地全局组。默认值:None。
use_calc_stream (bool,可选) - 标识使用计算流还是通信流。默认值:True,表示用计算流。
返回¶
Tensor, 从所有expert接收的数据。
代码示例¶
# required: distributed
import numpy as np
import paddle
from paddle.distributed import init_parallel_env
init_parallel_env()
n_expert = 2
world_size = 2
d_model = 2
in_feat = d_model
local_input_buf = np.array([[1, 2],[3, 4],[5, 6],[7, 8],[9, 10]], dtype=np.float32)
if paddle.distributed.ParallelEnv().local_rank == 0:
local_count = np.array([2, 1, 1, 1])
global_count = np.array([2, 1, 1, 1])
else:
local_count = np.array([1, 1, 2, 1])
global_count = np.array([1, 1, 2, 1])
local_input_buf = paddle.to_tensor(local_input_buf, dtype="float32", stop_gradient=False)
local_count = paddle.to_tensor(local_count, dtype="int64")
global_count = paddle.to_tensor(global_count, dtype="int64")
a = paddle.distributed.utils.global_gather(local_input_buf, local_count, global_count)
print(a)
# out for rank 0: [[1, 2], [3, 4], [7, 8], [1, 2], [7, 8]]
# out for rank 1: [[5, 6], [9, 10], [3, 4], [5, 6], [9, 10]]
a.stop_gradient = False
c = a * a
c.backward()
print("local_input_buf.grad", local_input_buf.grad)
# out for rank 0: [[2, 4], [6, 8], [10, 12], [14, 16], [18, 20]]
# out for rank 1: [[2, 4], [6, 8], [10, 12], [14, 16], [18, 20]]